Table of Contents
Introduction
Machine learning has become an increasingly important field in the world of technology and data analysis. Companies and researchers alike are harnessing the power of machine learning to optimize their processes and improve performance. In this article, we will explore 10 machine learning hacks that can help you achieve better results in your projects. From advanced techniques to troubleshooting common issues, we will cover a wide range of topics to enhance your machine learning skills.
Understanding Machine Learning Hacks
Before diving into the specific hacks, it is essential to have a solid understanding of the fundamentals of machine learning. We will discuss the basic concepts and principles behind machine learning, including the different types of learning algorithms and their applications. This section will provide a solid foundation for the subsequent hacks.
Advanced Techniques for Machine Learning Optimization
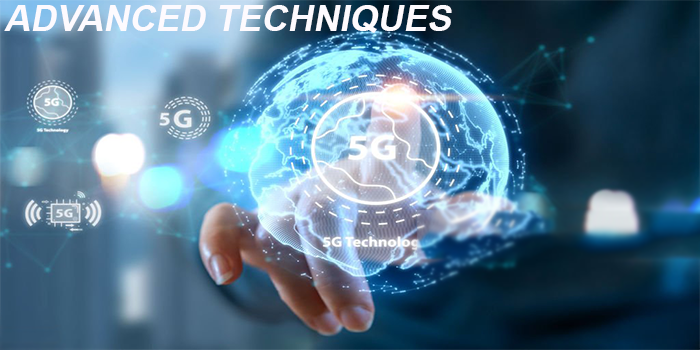
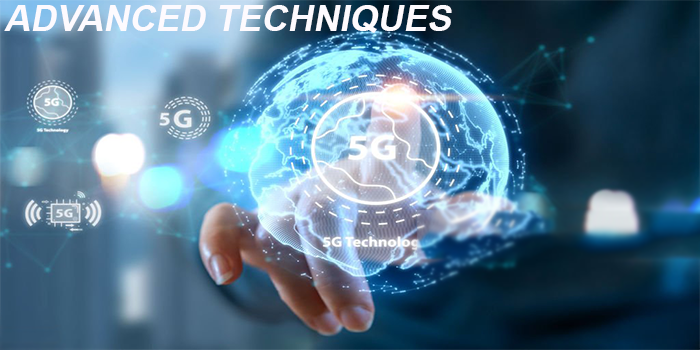
To truly optimize performance in machine learning, one must go beyond the basics and explore advanced techniques. This section will delve into various approaches for optimizing machine learning models, such as hyperparameter tuning, model ensemble, and regularization. By implementing these advanced techniques, you can significantly improve the accuracy and efficiency of your models.
Data Preprocessing in Machine Learning
Data preprocessing plays a crucial role in the success of machine learning models. This section will explore different techniques for cleaning and preparing data before feeding it into the models. We will discuss handling missing values, dealing with outliers, and scaling features to ensure accurate and reliable results.
Feature Engineering in Machine Learning
Feature engineering involves transforming raw data into meaningful features that can enhance the performance of machine learning models. We will explore various feature engineering techniques, such as dimensionality reduction, one-hot encoding, and feature selection. By effectively engineering features, you can extract more valuable information from your data, leading to improved model performance.
Exploring Different Machine Learning Algorithms
Machine learning offers a diverse range of algorithms, each suited for different types of problems. In this section, we will explore popular supervised and unsupervised machine learning algorithms, such as decision trees, support vector machines, clustering algorithms, and neural networks. Understanding the strengths and weaknesses of each algorithm will empower you to choose the most appropriate one for your specific task.
Supervised Machine Learning
Supervised learning is a common approach in machine learning where models are trained using labeled data. In this section, we will discuss different supervised learning techniques, including linear regression, logistic regression, and k-nearest neighbors. We will cover the training process and evaluation metrics to measure the performance of these models accurately.
Unsupervised Machine Learning
Unlike supervised learning, unsupervised learning involves training models without labeled data. Clustering and dimensionality reduction are some of the techniques used in unsupervised learning. This section will explore popular unsupervised learning algorithms, such as K-means clustering and principal component analysis (PCA), and illustrate how they can be applied to real-world scenarios.
Reinforcement Learning in Machine Learning
Reinforcement learning is a unique branch of machine learning where an agent learns from its interactions with an environment. This section will provide an overview of reinforcement learning algorithms, such as Q-learning and deep Q-networks. We will also discuss the applications of reinforcement learning in various fields, including robotics and game playing.
Transfer Learning in Machine Learning
Transfer learning is a technique that allows models to leverage existing knowledge from one task to improve performance on another related task. This section will explore transfer learning methods, including fine-tuning pre-trained models and using feature extraction. We will discuss how to effectively apply transfer learning in different scenarios to accelerate model development.
Building Effective Machine Learning Models
Creating effective machine learning models requires a combination of good practices and a deep understanding of the problem domain. This section will cover the essential steps involved in building robust models, including data splitting, cross-validation, and model evaluation. We will also discuss techniques for handling imbalanced datasets and ensuring model fairness.
Applications of Machine Learning
Machine learning has found its applications in various industries, from healthcare to finance and beyond. In this section, we will explore real-world use cases where machine learning has been successfully applied. Examples include fraud detection, recommendation systems, natural language processing, and image classification. Understanding these applications will inspire you to explore the vast possibilities of machine learning.
Exploring Machine Learning Frameworks
To facilitate the development and deployment of machine learning models, several frameworks have been developed. This section will introduce some popular machine learning frameworks, such as TensorFlow and PyTorch. We will discuss their features, advantages, and use cases, enabling you to choose the most suitable framework for your projects.
Utilizing Machine Learning Libraries
Machine learning libraries provide a range of tools and functions that simplify the implementation of complex algorithms. In this section, we will explore widely-used machine learning libraries such as scikit-learn, Pandas, and NumPy. We will showcase their capabilities and demonstrate how to leverage them effectively to accelerate your machine learning workflow.
Creating Machine Learning Pipelines
Machine learning pipelines help streamline the process of building and deploying models by automating repetitive tasks. This section will guide you through the creation of efficient pipelines using tools like Apache Airflow and Kubeflow. We will discuss best practices for pipeline design and deployment, enabling you to automate your end-to-end machine learning workflows.
Automation in Machine Learning
Automation is a key aspect of machine learning that can significantly improve efficiency and productivity. This section will explore different methods of automating machine learning tasks, such as hyperparameter optimization and model selection. We will discuss the role of AutoML platforms and how they simplify the machine learning process, even for users with limited technical expertise.
Deployment of Machine Learning Models
Deploying machine learning models into production involves several challenges, including scalability, robustness, and integration with existing systems. In this section, we will discuss different deployment strategies, such as using APIs or containerization, and explore platforms like TensorFlow Serving and AWS SageMaker. By understanding the deployment process, you can ensure your models are ready for real-world use.
Improving Machine Learning Performance
Optimizing machine learning performance goes beyond just training models. This section will cover techniques for improving model speed and efficiency, such as parallel computing and model compression. We will discuss hardware acceleration using GPUs and TPUs, and how to make your models more resource-efficient without sacrificing accuracy.
Troubleshooting Machine Learning Issues
Machine learning projects often face challenges such as overfitting, underfitting, and data leakage. This section will provide troubleshooting strategies to overcome these issues and ensure reliable model performance. We will discuss techniques like regularization, cross-validation, and data augmentation to tackle common problems encountered during model development.
Best Practices for Machine Learning
Adhering to best practices is crucial for successful machine learning projects. This section will cover essential guidelines for data collection, annotation, and model evaluation. We will also discuss the importance of data ethics and bias mitigation, ensuring that your machine learning solutions are fair and unbiased.
Tips and Tricks for Machine Learning
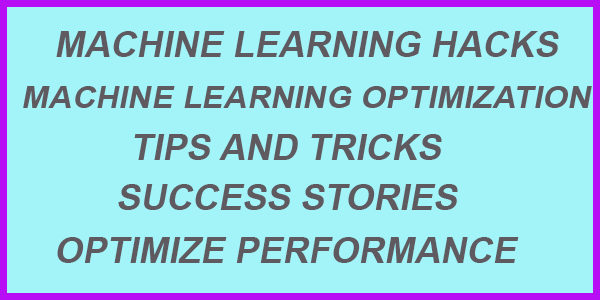
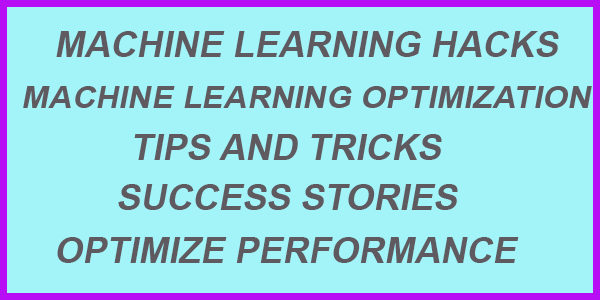
In this section, we will share valuable tips and tricks that can boost your productivity and enhance the performance of your machine learning models. From utilizing early stopping to leveraging ensemble methods, these tips will help you navigate the intricacies of machine learning and achieve better results in less time.
Strategies for Machine Learning Success
Achieving success in machine learning requires careful planning and execution. This section will provide strategies for effectively approaching machine learning projects, including setting clear goals, defining metrics, and conducting thorough evaluations. By adopting a strategic mindset, you can maximize the impact of your machine learning endeavors.
Conducting Machine Learning Experiments
Conducting experiments is an essential part of the machine learning process. This section will guide you through the entire lifecycle of a machine learning experiment, including data setup, model development, and evaluation. We will discuss methodologies like A/B testing and explain how to draw meaningful conclusions from your experimental results.
Case Studies in Machine Learning
Real-world case studies offer valuable insights into the practical application of machine learning. In this section, we will explore several case studies that highlight successful machine learning implementations across different industries. By examining these examples, you can gain inspiration and learn from the experiences of others.
Success Stories in Machine Learning
Success stories in machine learning serve as motivation and inspiration for aspiring data scientists. This section will showcase notable success stories where machine learning has driven groundbreaking advancements or solved complex problems. From self-driving cars to medical diagnostics, these stories will emphasize the transformative potential of machine learning.
In conclusion, these 10 machine learning hacks, ranging from advanced techniques to deployment strategies, can greatly optimize the performance of your machine learning projects. By understanding the key concepts, implementing best practices, and leveraging powerful tools and frameworks, you can unlock the full potential of machine learning and drive impactful results.